Linear Regression¶

Learns a linear function of its input data.
Signals¶
Inputs:
Data
A data set
Preprocessor
A preprocessed data set.
Outputs:
Learner
A learning algorithm with the supplied parameters
Predictor
A trained regressor. Signal Predictor sends the output signal only if signal Data is present.
Description¶
The Linear Regression widget constructs a learner/predictor that learns a linear function from its input data. The model can identify the relationship between a predictor xi and the response variable y. Additionally, Lasso and Ridge regularization parameters can be specified. Lasso regression minimizes a penalized version of the least squares loss function with L1-norm penalty and Ridge regularization with L2-norm penalty.
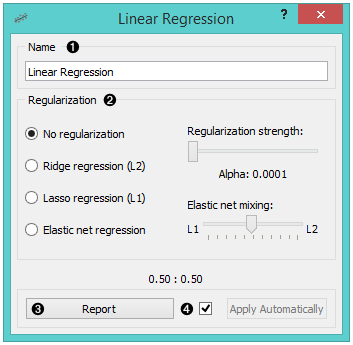
- The learner/predictor name
- Choose a model to train:
- no regularization
- a Ridge regularization (L2-norm penalty)
- a Lasso bound (L1-norm penalty)
- an Elastic net regularization
- Produce a report.
- Press Apply to commit changes. If Apply Automatically is ticked, changes are committed automatically.
Example¶
Below, is a simple workflow showing how to use both the Predictor and the Learner output. We used the Housing data set. For the Predictor, we input the prediction model into the Predictions widget and view the results in the Data Table. For the Learner, we can compare different learners in the Test&Score widget.
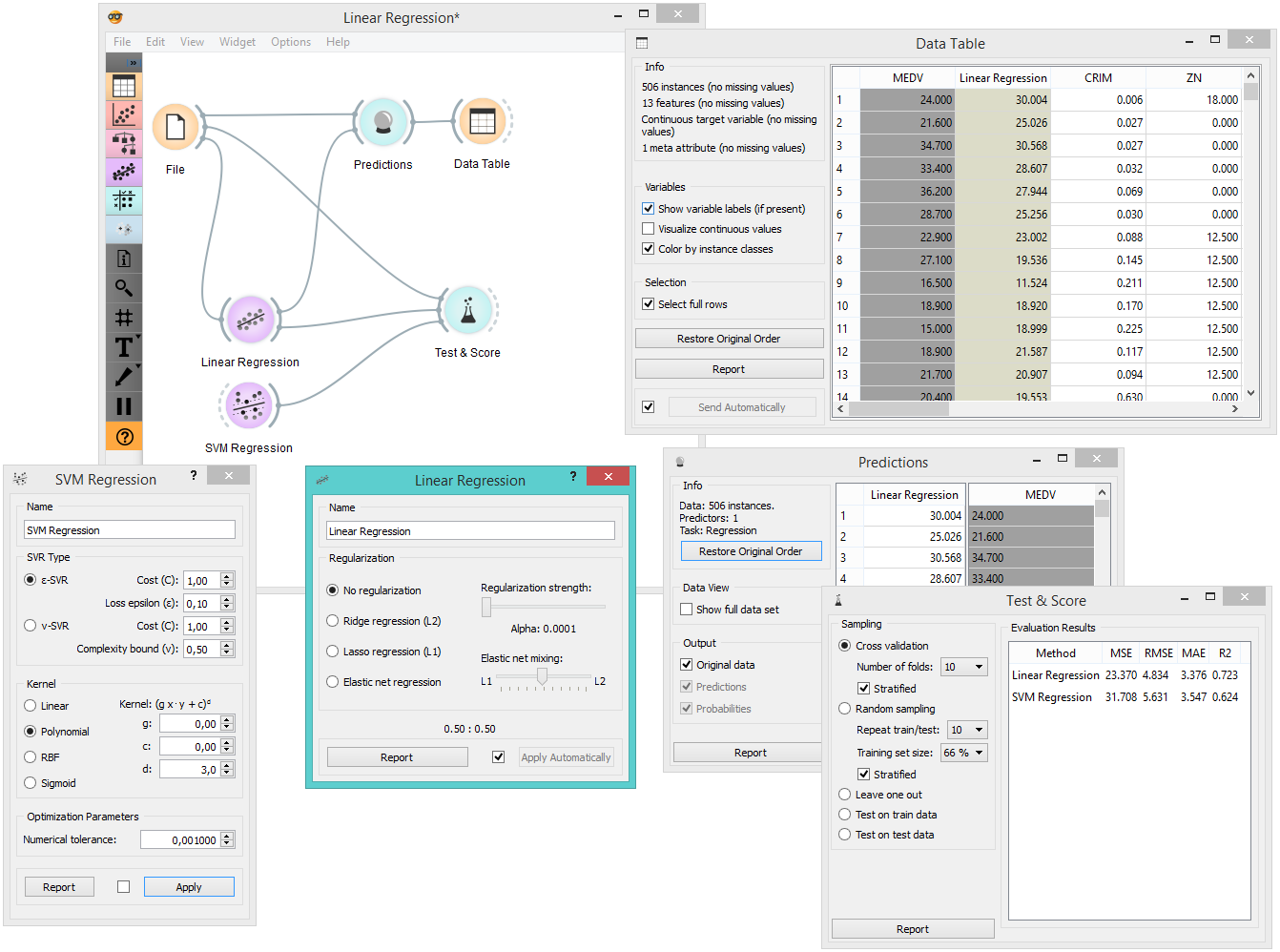